papers on AI and computation
Artificial Minds
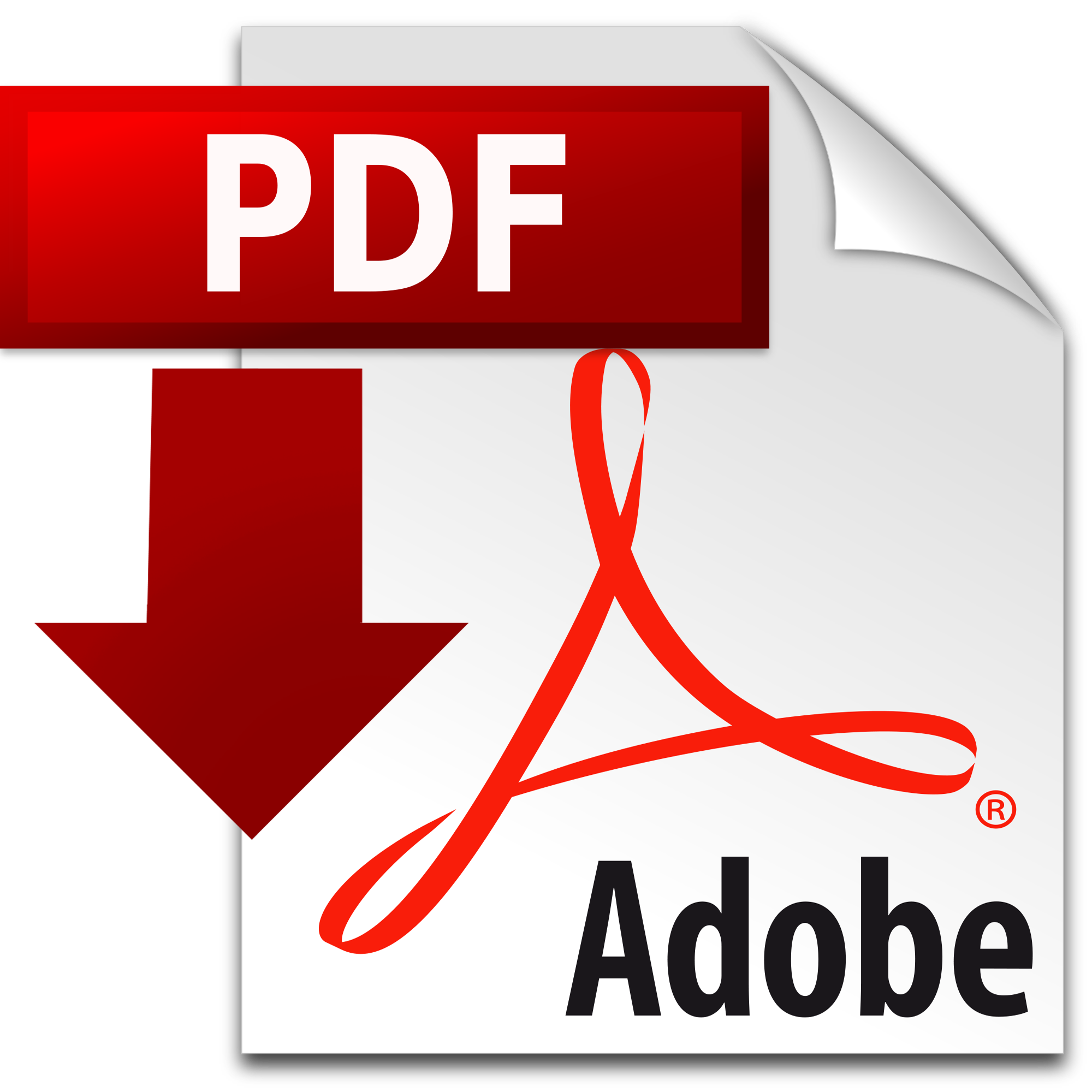
The Singularity: A Philosophical Analysis (2010)
Journal of Consciousness Studies 17:7-65, 2010. A long article on artificial superintelligence. I try to make the argument for a rapid “intelligence explosion” philosophically rigorous. There’s also some discussion of AI safety issues and mind uploading. There were subsequently 26 commentaries and my reply below. [pdf]
[philpapers]
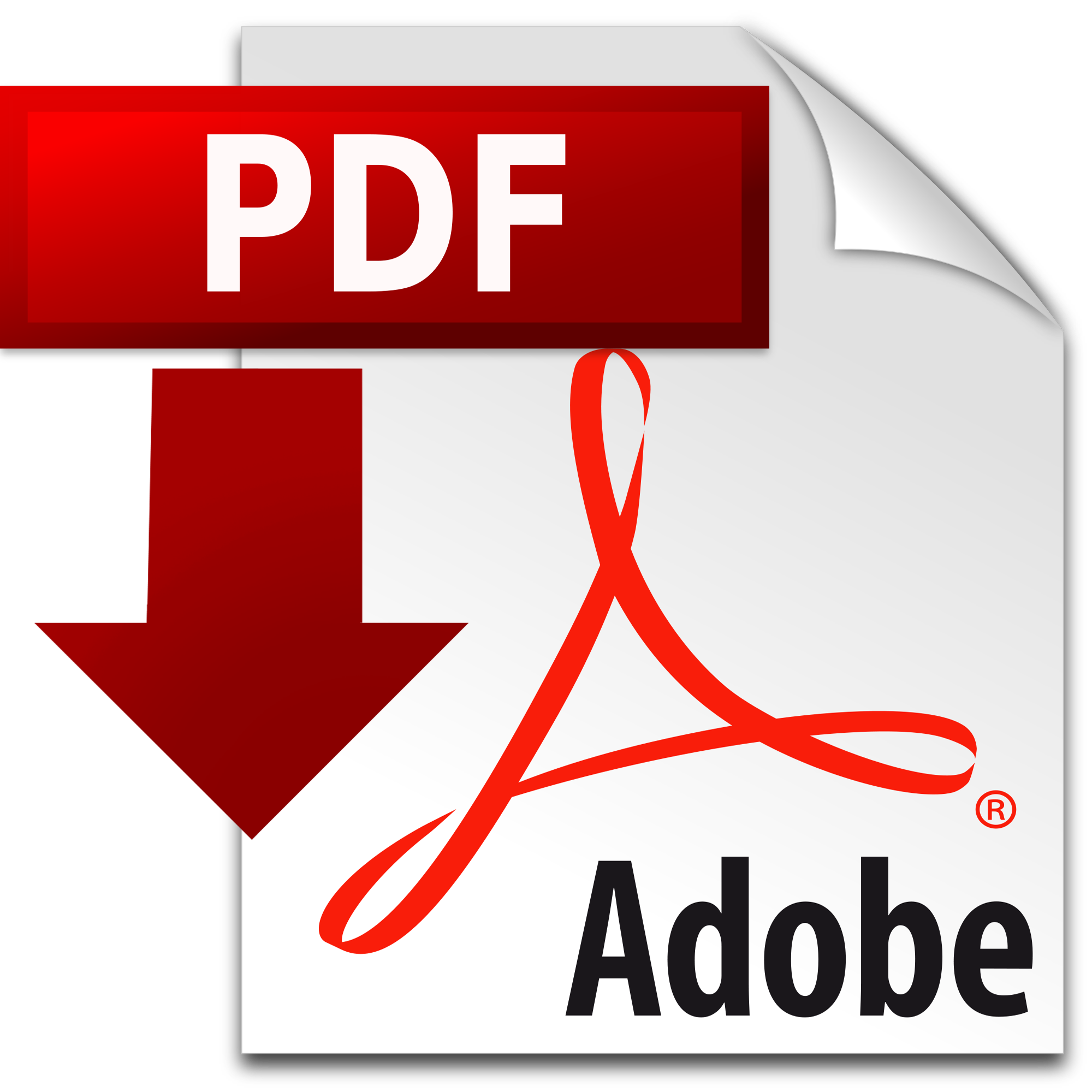
The Singularity: A Reply (2012)
Journal of Consciousness Studies 19:141-67, 2012. This was my reply to 26 commentaries by Sue Blackmore, Nick Bostrom, Barry Dainton, Dan Dennett, Robin Hanson, Marcus Hutter, Ray Kurzweil, Drew McDermott, Jesse Prinz, Susan Schneider, Jurgen Schmidhuber, and others. This is my reply in turn. The whole symposium was published as a special issue of the journal and later as the oddly-subtitled book The Singularity: Could artificial intelligence really out-think us (and would we want it to) from Imprint Academic. For what it’s worth I think the argument for an intelligence explosion holds up pretty well. [pdf] [philpapers]
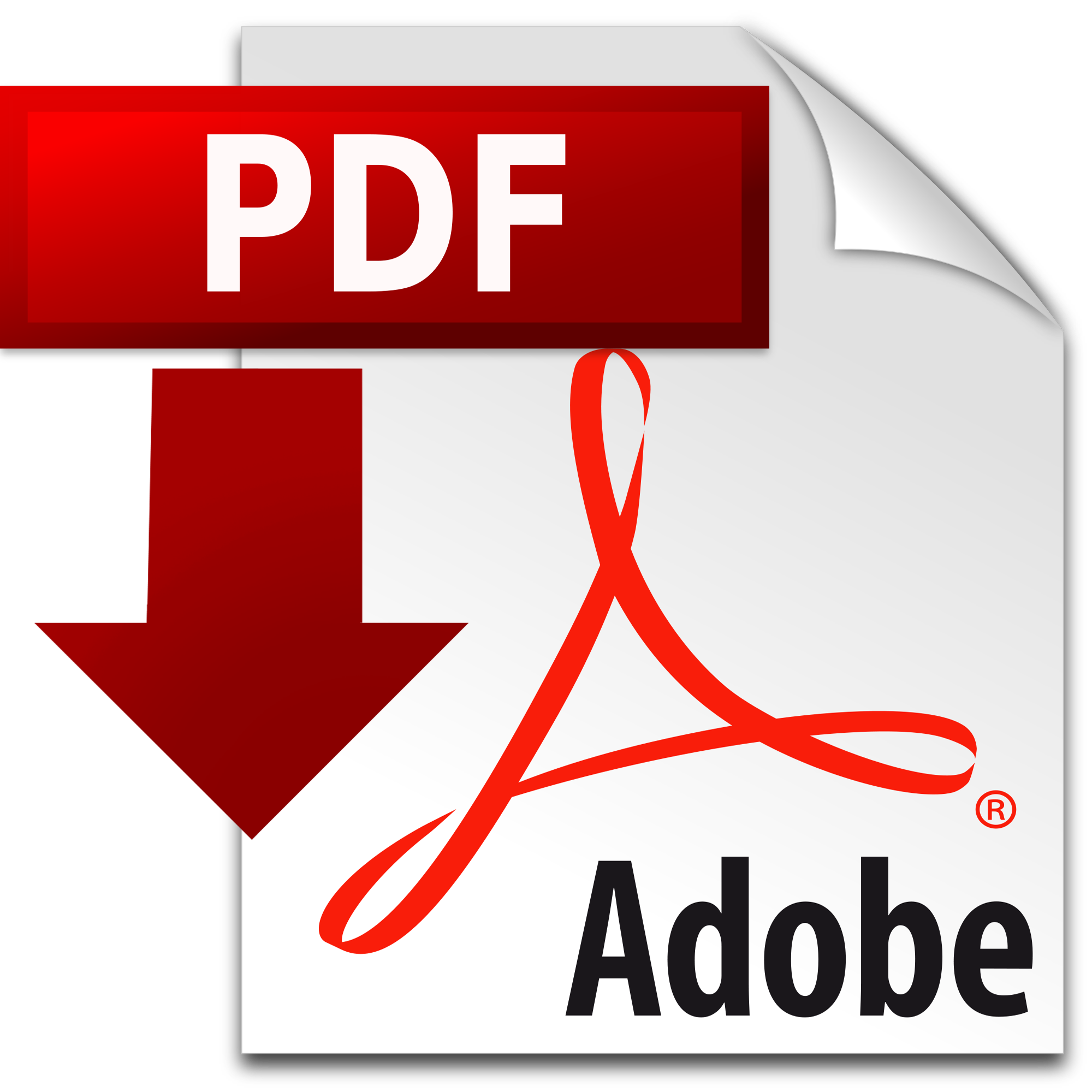
Mind Uploading: A Philosophical Analysis (2014)
In (Russell Blackford and Damien Broderick, eds.) Intelligence Unbound: The Future of Uploaded and Machine Minds (Wiley-Blackwell, 2014). A discussion of philosophical issues regarding uploading our minds into computers. Originally this was published as the last one-third of the singularity article above, and later it was split off as a separate article for Blackford/Broderick collection. I think this is more or less the first time I’ve written about personal identity. [pdf]
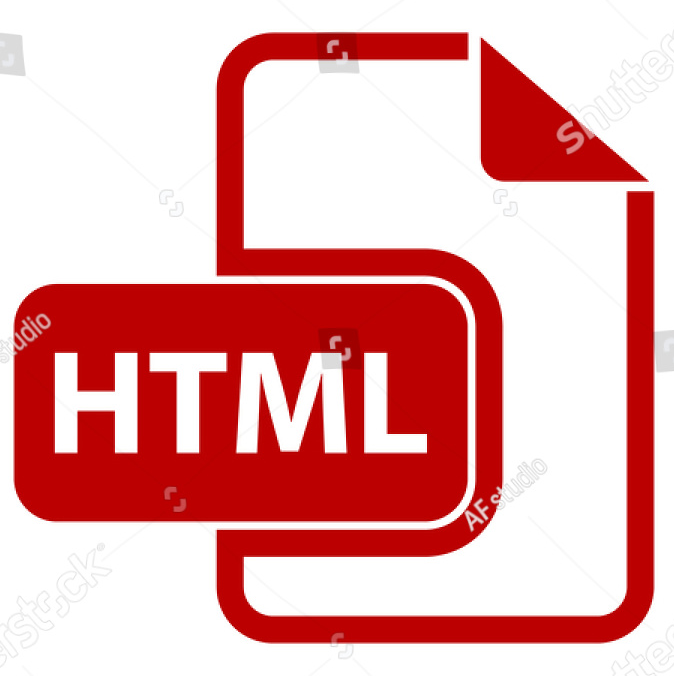
Minds, Machines, and Mathematics (1996)
This is a commentary on Roger Penrose’s book Shadows of the
Mind, focusing on his attempt to use Gödel’s theorem to
demonstrate the noncomputability of thought. I argue that the attempt is ultimately unsuccessful, but that there is a novel argument here that many commentators have overlooked, and that it raises many interesting issues. I also comment on his proposals concerning “the missing science of consciousness”. This paper appears in PSYCHE,2:11-20, 1995 in a symposium on Penrose’s book. Penrose replied in “Beyond the Doubting of a Shadow”. [html] [philpapers]
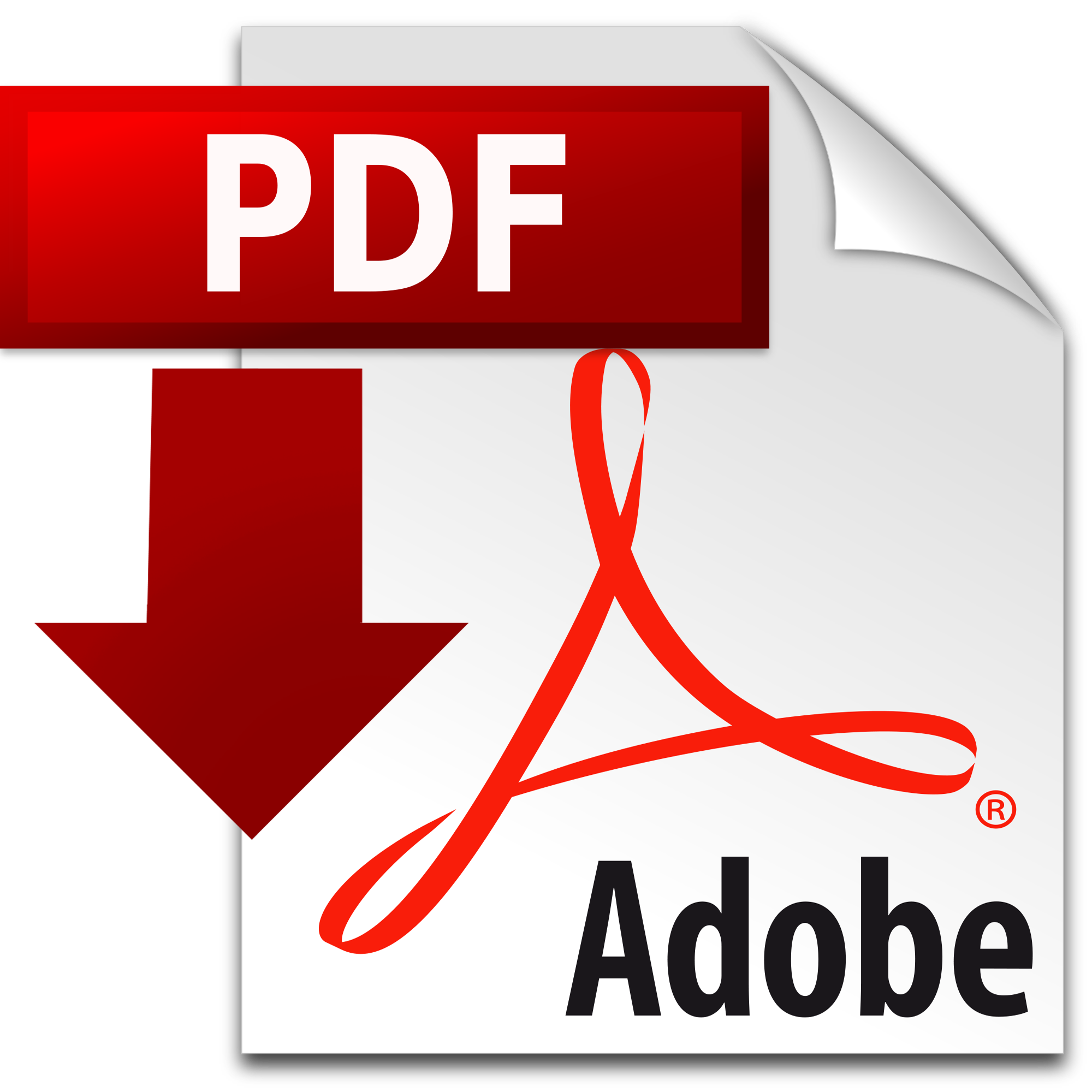
Computing the Thinkable (1990)
Behavioral and Brain Sciences 13: 658-9, 1990. This is a commentary in a BBS symposium on Roger Penrose’s book The Emperor’s New Mind.
Virtual Reality and Simulated Worlds
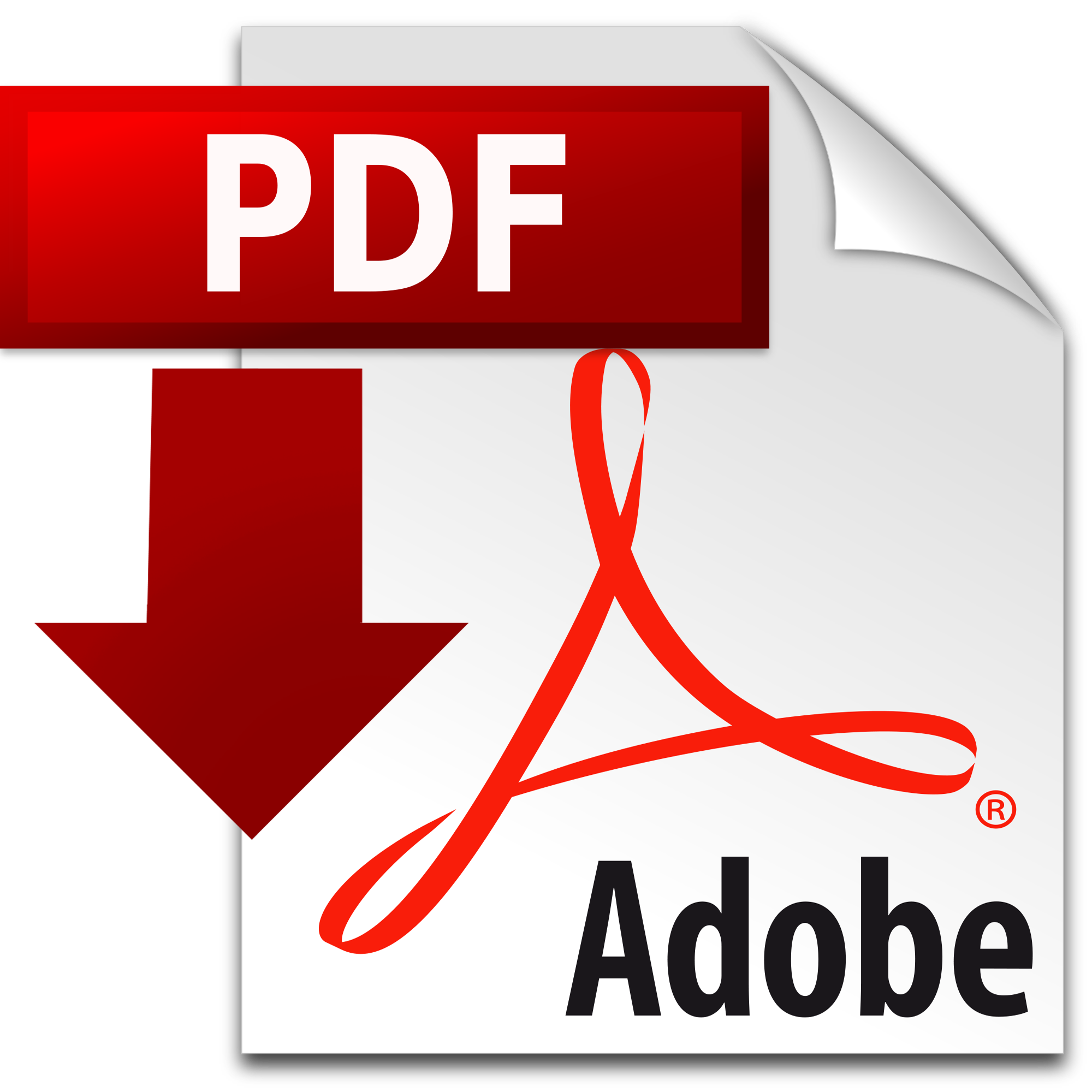
The Matrix as Metaphysics (2003)
First published on thematrix.com in 2003, and printed in (C. Grau, ed) Philosophers Explore the Matrix (Oxford University Press, 2005). I argue that even if we are in a Matrix-style simulation, most of our ordinary beliefs about the world are true. The hypothesis that we are in a Matrix is really a metaphysical hypothesis: one according to which physical objects are constituted by computations and all this was designed by a designer (in the next world up). I use this to argue against a sort of Cartesian global skepticism about the external world. [pdf] [philpapers]
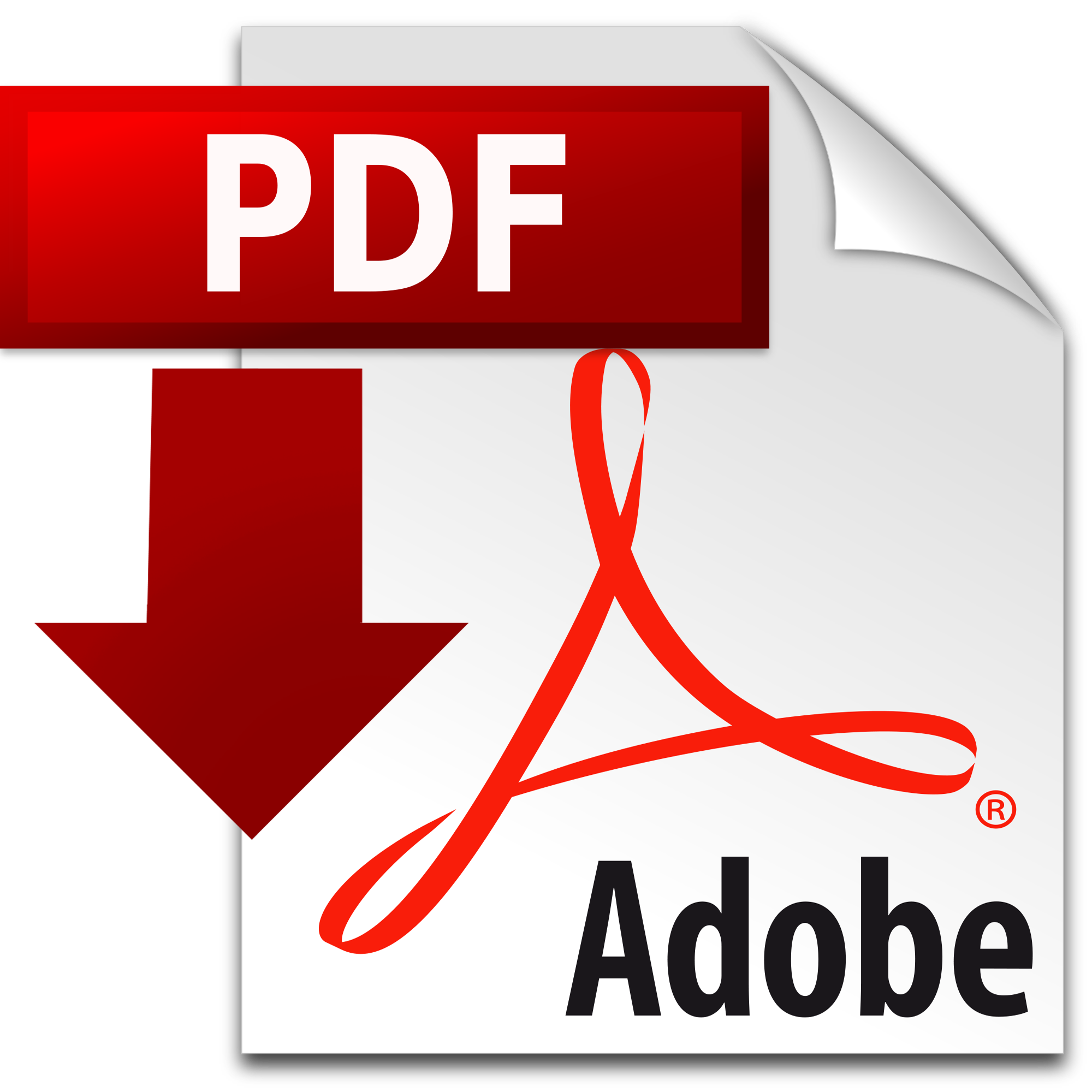
The Virtual and the Real (2017)
Disputatio 9(46):309-352. On the metaphysics of virtual reality. I defend a sort of virtual realism and virtual digitalism (on which virtual objects are real digital objects)
over virtual irrealism and virtual fictionalism (where virtual objects are fictional objects). With discussion of the definition of VR, of illusions in VR, of the value of VR and Nozick’s Experience Machine, of augmented reality and dreams, of the connection to structuralism and skepticism, and more. This paper is the subject of a symposium on Disputatio with a number of commentaries and a reply. [pdf] [philpapers]
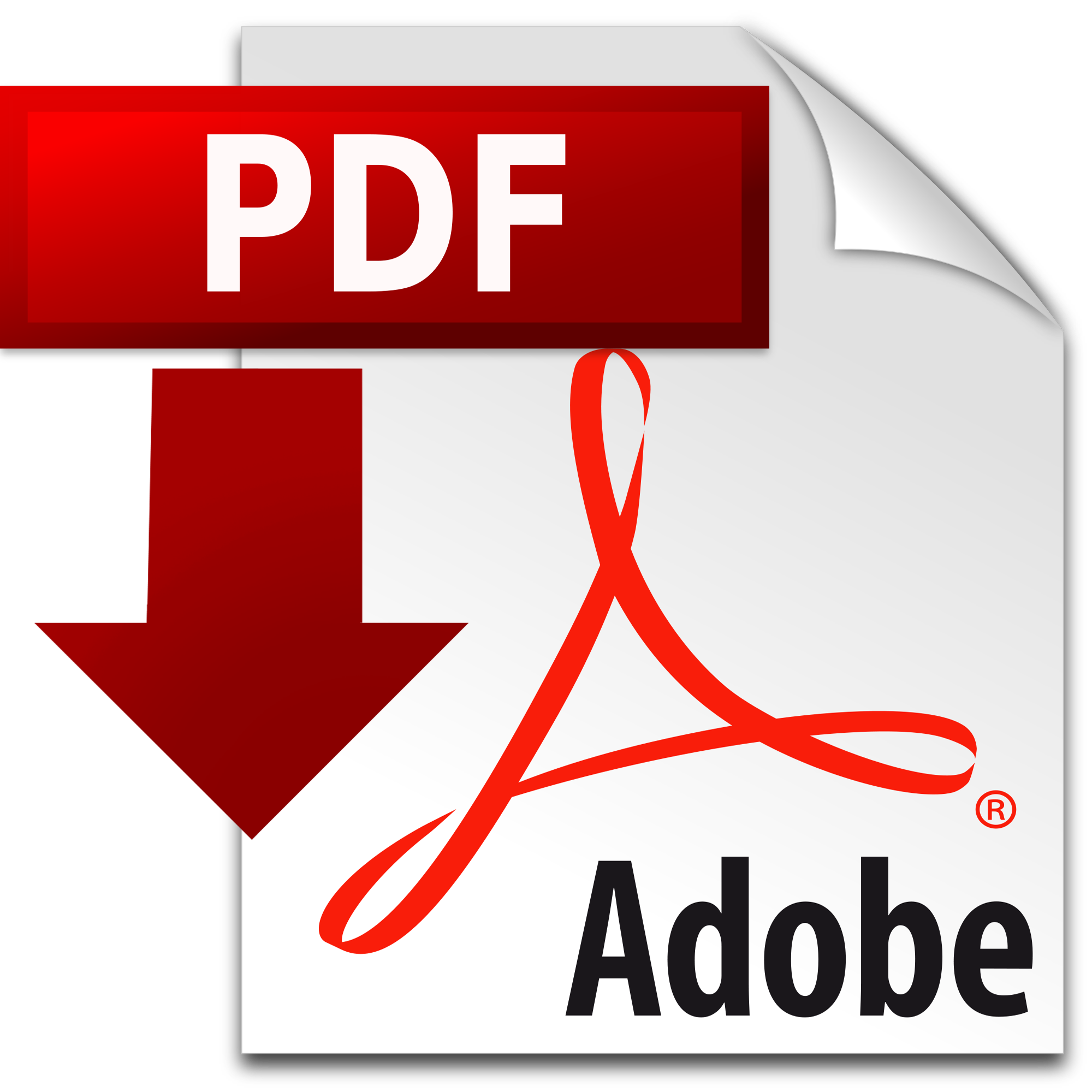
The Virtual as the Digital (2020)
Disputatio, 2020. A reply to seven commentaries on “The Virtual and the Real”, focusing especially on virtual objects as digital objects, and on issues about space in virtual worlds. [pdf] [philpapers]
Computation in Physical Systems
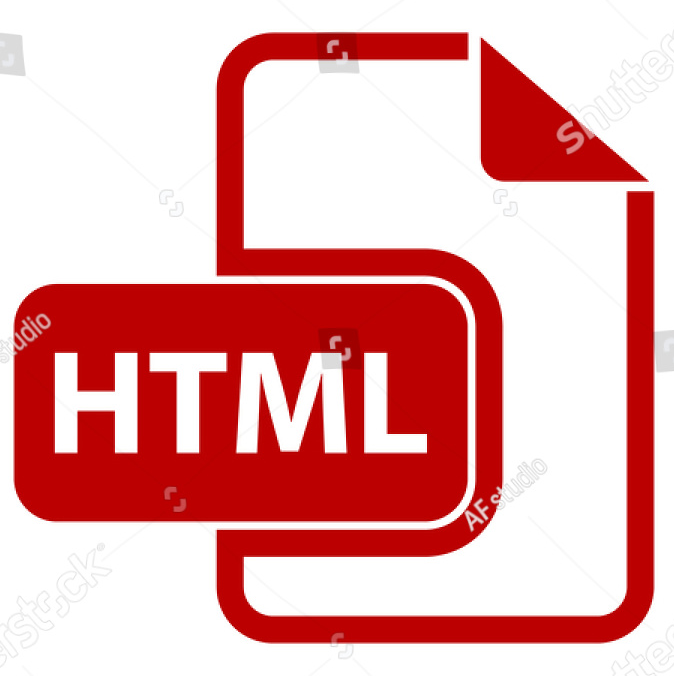
A Computational Foundation for the Study of Cognition (1995/2012)
This paper addresses some key questions about computation and its
role in cognitive science. I give an account of what it takes for a
physical system to implement a given computation (in terms of abstract
patterns of causal organization), and use this account to defend
“strong artificial intelligence” and justify the centrality
of computational explanation in cognitive science. This paper was
written in 1993 but unpublished for many years (though section 2
appeared in “On Implementing a Computation” in Minds and
Machines, 1994), and was finally published as the subject of a
2012 symposium in the Journal of Cognitive Science. [html] [philpapers].
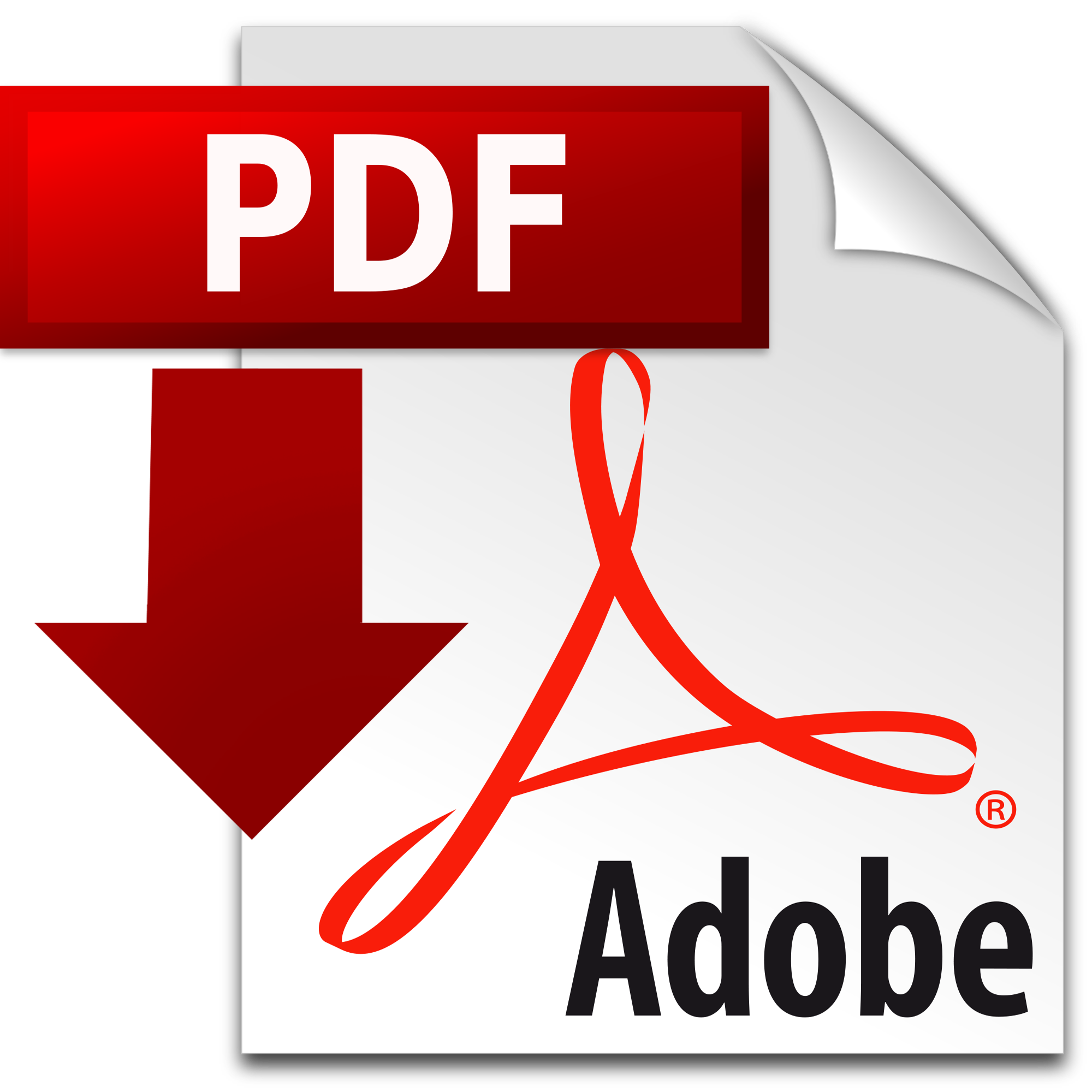
The Varieties of Computation: A Reply (2012)
Journal of Cognitive Science. A reply to comments by Curtis Brown, Frances Egan, Stevan Harnad, Colin Klein, Gerard O’Brien, Marcin Milkowski, Brendan Ritchie, Michael Rescorla, Matthias Scheutz, Oron Shagrir, Mark Sprevak, Brandon Towl. With discussion of pluralism about computation, computation and representation, and of counterexamples of structuralist accounts of implementation. [pdf] [philpapers]
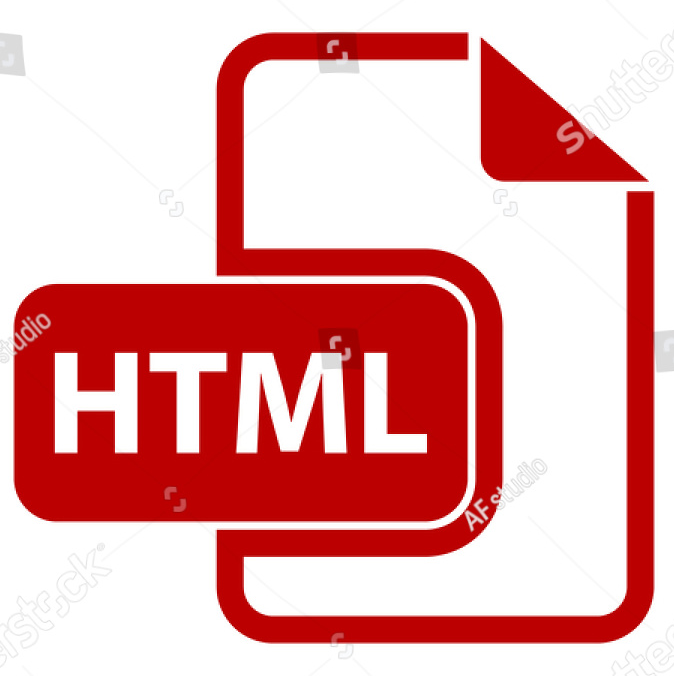
Does A Rock Implement Every Finite-State Automaton? (1996)
In an appendix to his book Representation and Reality, Hilary
Putnam “proves” that every ordinary open system implements every
finite automaton, so that computation cannot provide a nonvacuous foundation
for the sciences of the mind. I analyze Putnam’s argument and find it wanting. The argument can be patched up to some extent, but this only points the way to a better definition of implementation (of combinatorial-state automata) that is invulnerable to such an objection. A couple of open questions remain, however. Synthese 108: 309-33, 1996. [html] [philpapers]
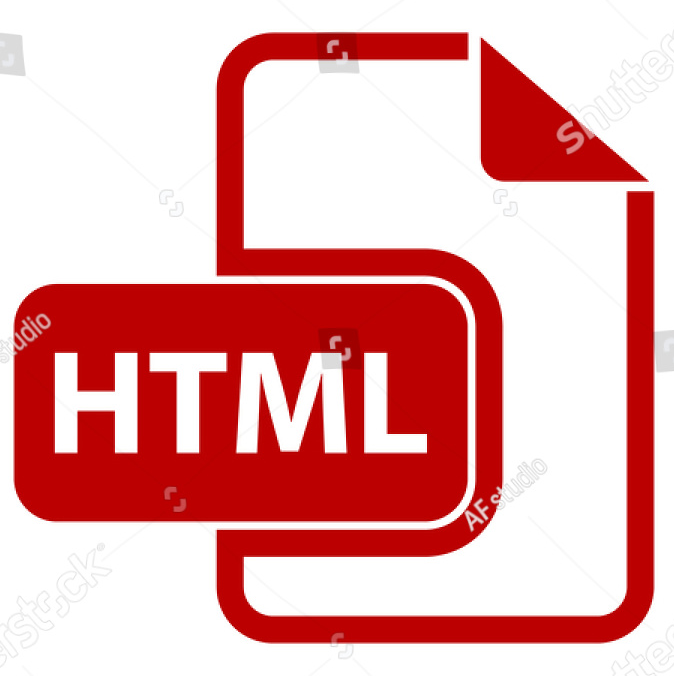
On Implementing a Computation (1994)
Minds and Machines, 1994. Pro-tip: This paper is more or less a proper subset of “>”A Computational Foundation for the Study of Cognition”. [html] [philpapers]
Connectionism and Subsymbolic Computation
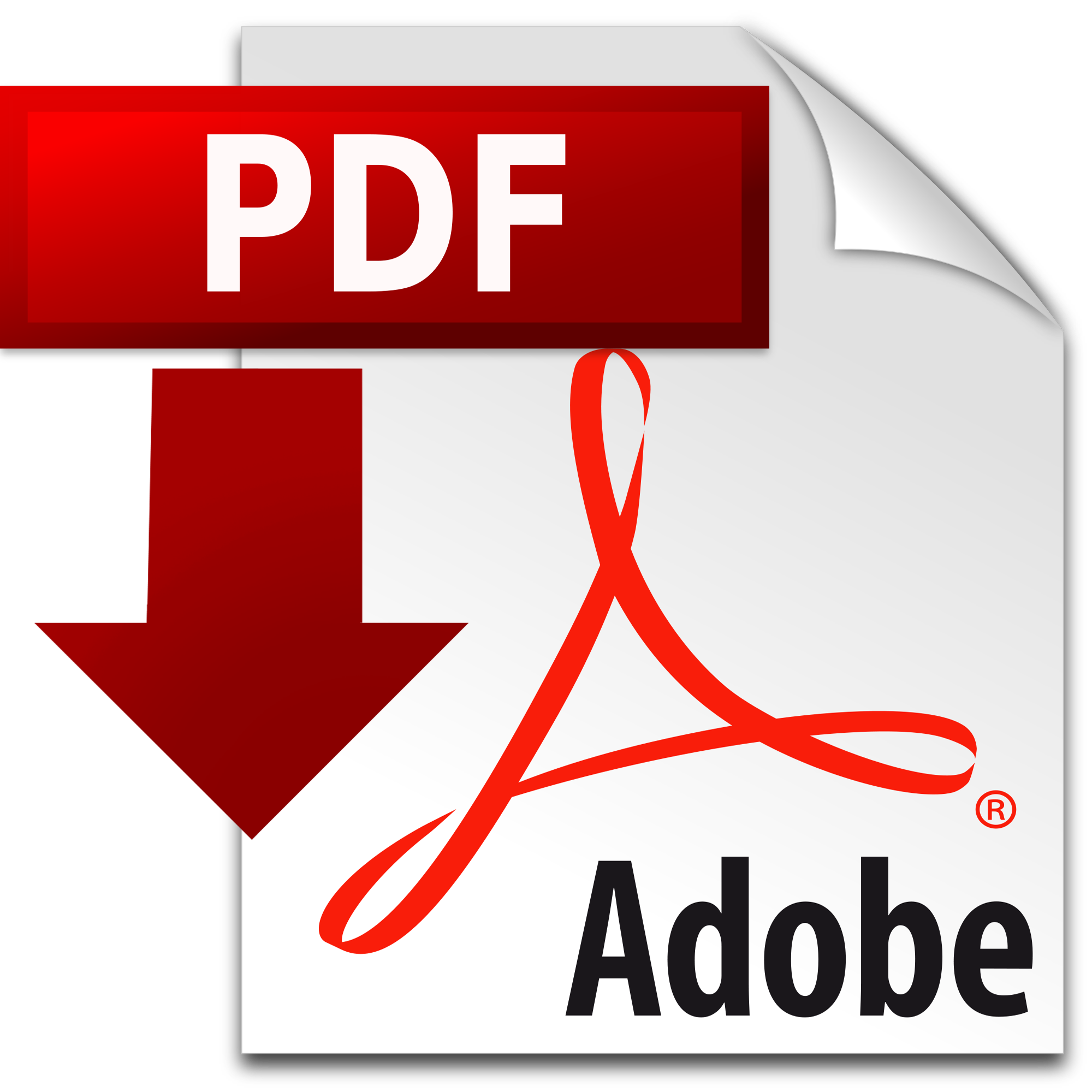
High-Level Perception, Analogy, and Representation: A Critique of Artificial Intelligence Methodology
Co-authored with Bob French and Doug Hofstadter. Journal of Experimental and Theoretical Artificial Intelligence 4:185-211, 1992. Reprinted in (D. R. Hofstadter) Fluid Concepts and Creative Analogies. Basic Books. This paper argues that high-level perception is crucially involved in most cognitive processing. We mount a critique of the common approach of using “frozen”, hand-coded representations in cognitive modeling (exemplified by Langley and Simon’s BACON and Gentner’s Structure-Mapping Engine), and argue for a different approach in which representations are constructed and molded “on the fly”. There was a response by Forbus, Gentner, Markman, and Ferguson, as well as a commentary by Morrison and Dietrich. [pdf] [philpapers]
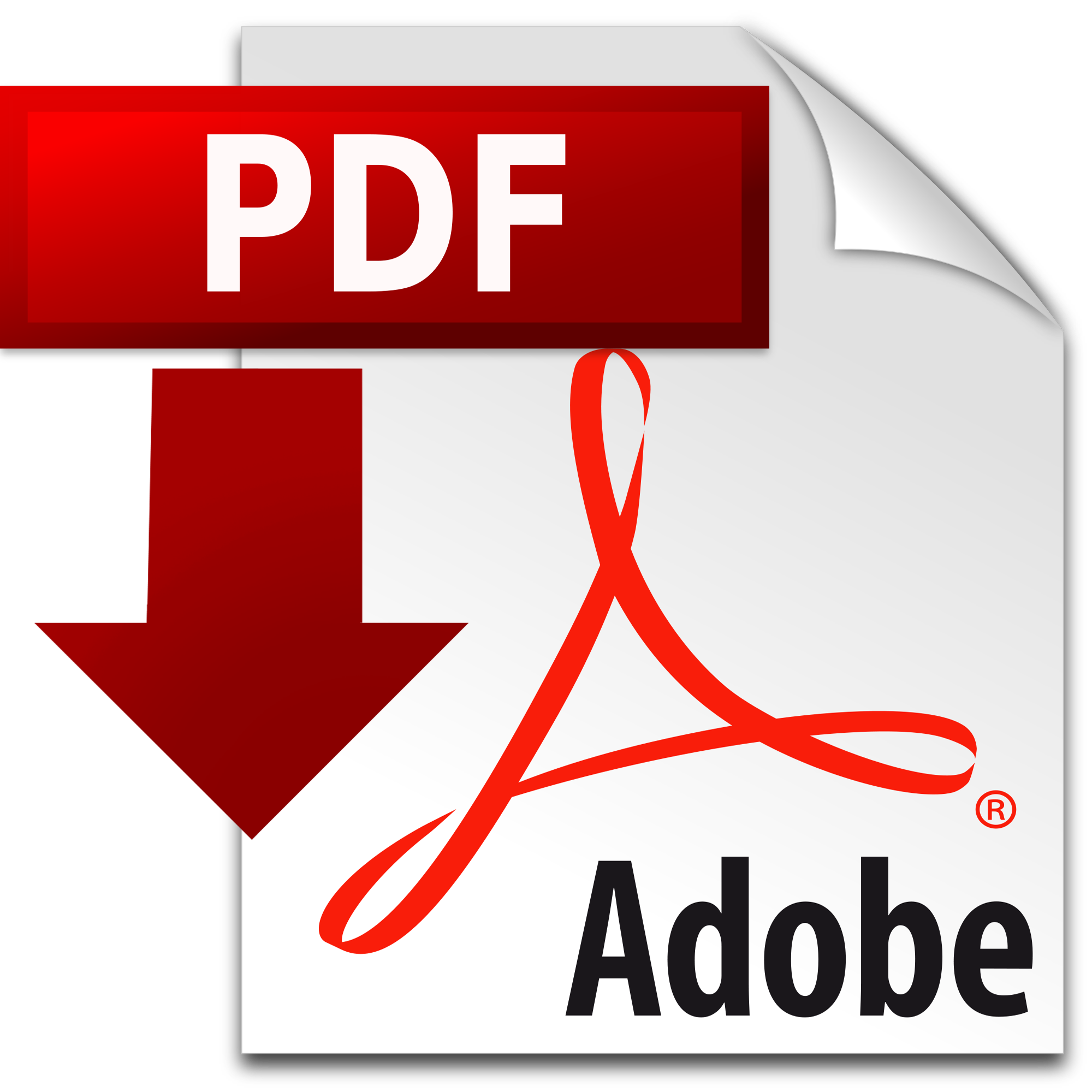
Syntactic Transformations on Distributed Representations (1990)
In this paper I demonstrate that a connectionist network can be used
to perform systematic structure-sensitive transformations on compressed
distributed representations of compositional structures. Using representations developed by a Recursive Auto-Associative Memory (a model of Jordan Pollack‘s), a feedforward network learns to move systematically from representations of an active sentence to that of a corresponding passive sentence, and vice versa. This paper appeared in Connection Science in 1990. This line of research has since been extended by a number of others, e.g. in Lonnie Chrisman’s “Learning
Recursive Distributed Representations for Holistic Computation”. [pdf] [philpapers]
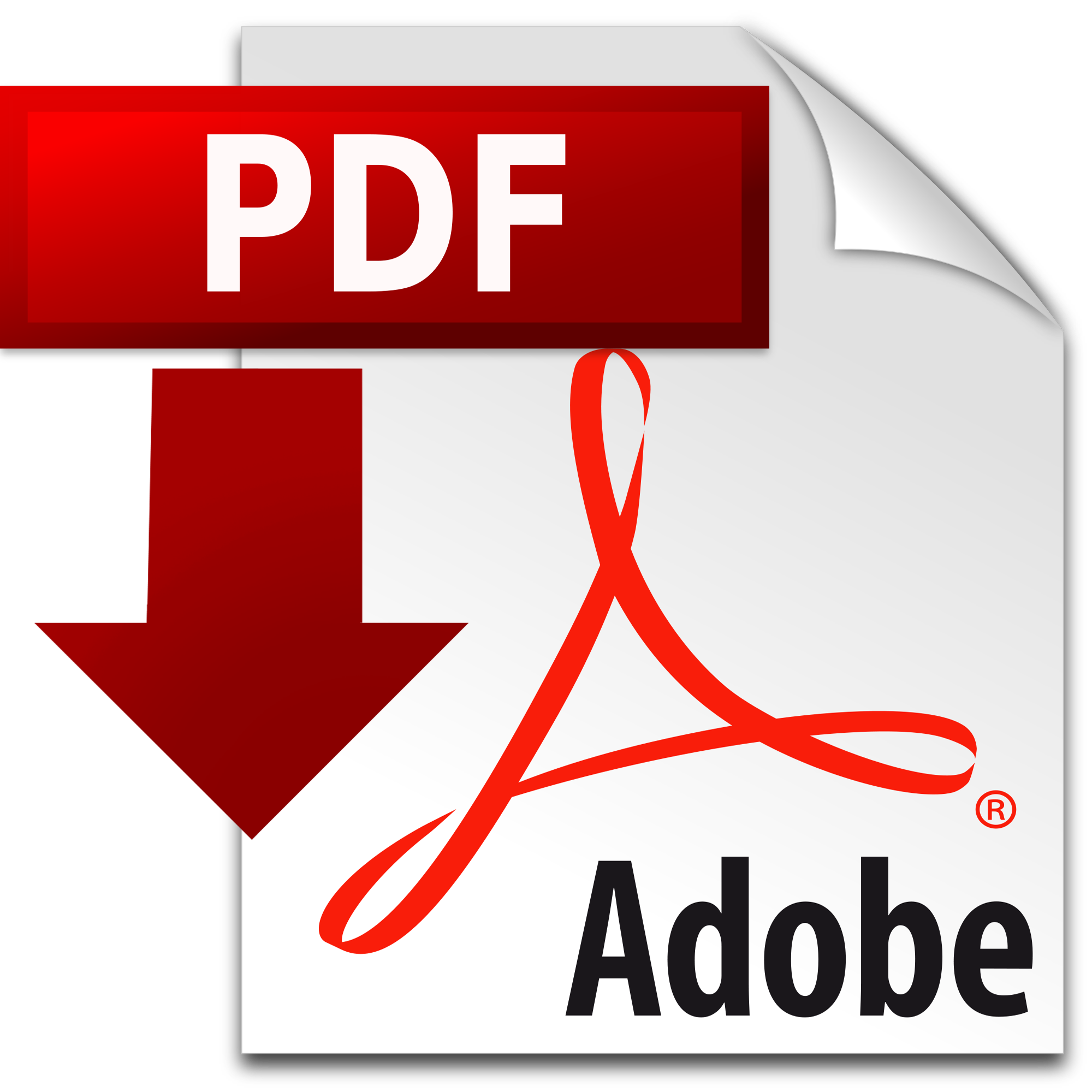
Connectionism and Compositionality: Why Fodor and Pylyshyn Were Wrong (1993)
I point out some structural problems with Fodor and Pylyshyn’s arguments
against connectionism, and trace these to an underestimation of the role
of distributed representation. I discuss some empirical results (from the
paper above) that have some bearing on Fodor and Pylyshyn’s argument. This
paper was published in Philosophical Psychology in 1993 (an earlier version was in the 1990 Proceedings of the Cognitive Science Society). See Murat Aydede’s “Connectionism and the Language of Thought” for some discussion. [pdf] [philpapers]
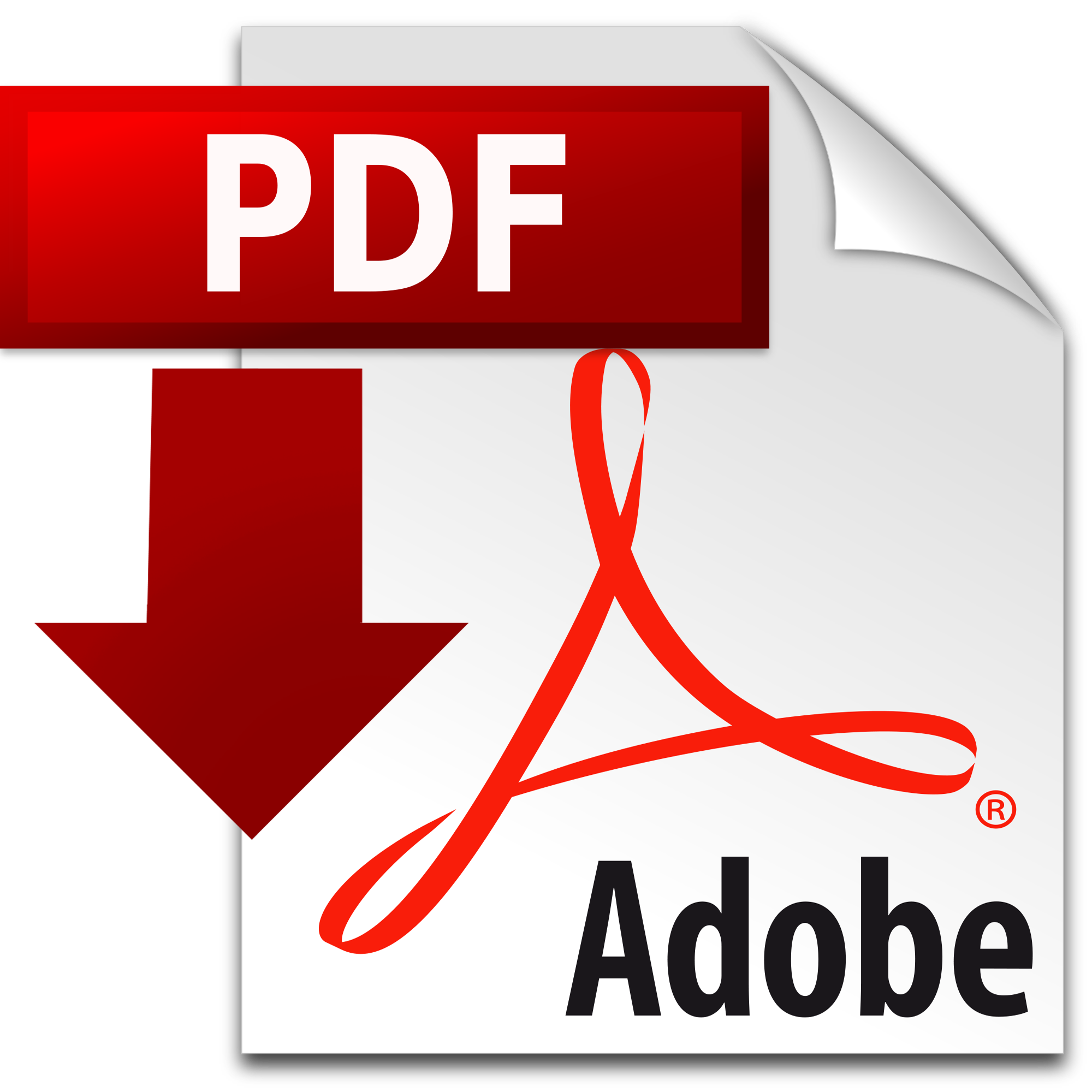
The Evolution of Learning: An Experiment in Genetic Connectionism (1990)
I combine genetic algorithms and neural networks to show how learning
mechanisms might evolve in a population of organisms that initially have
no capacity to learn. The dynamics of a neural network’s cross-time development are specified in a genome, and phenotypes are selected for their ability to learn various tasks across a lifetime. Over many generations, sophisticated learning mechanisms are developed, including on occasion the well-known delta rule. This paper appeared in the Proceedings of the 1990 Connectionist Summer School Workshop. See here
for more models of evolution and learning. [pdf] [philpapers]
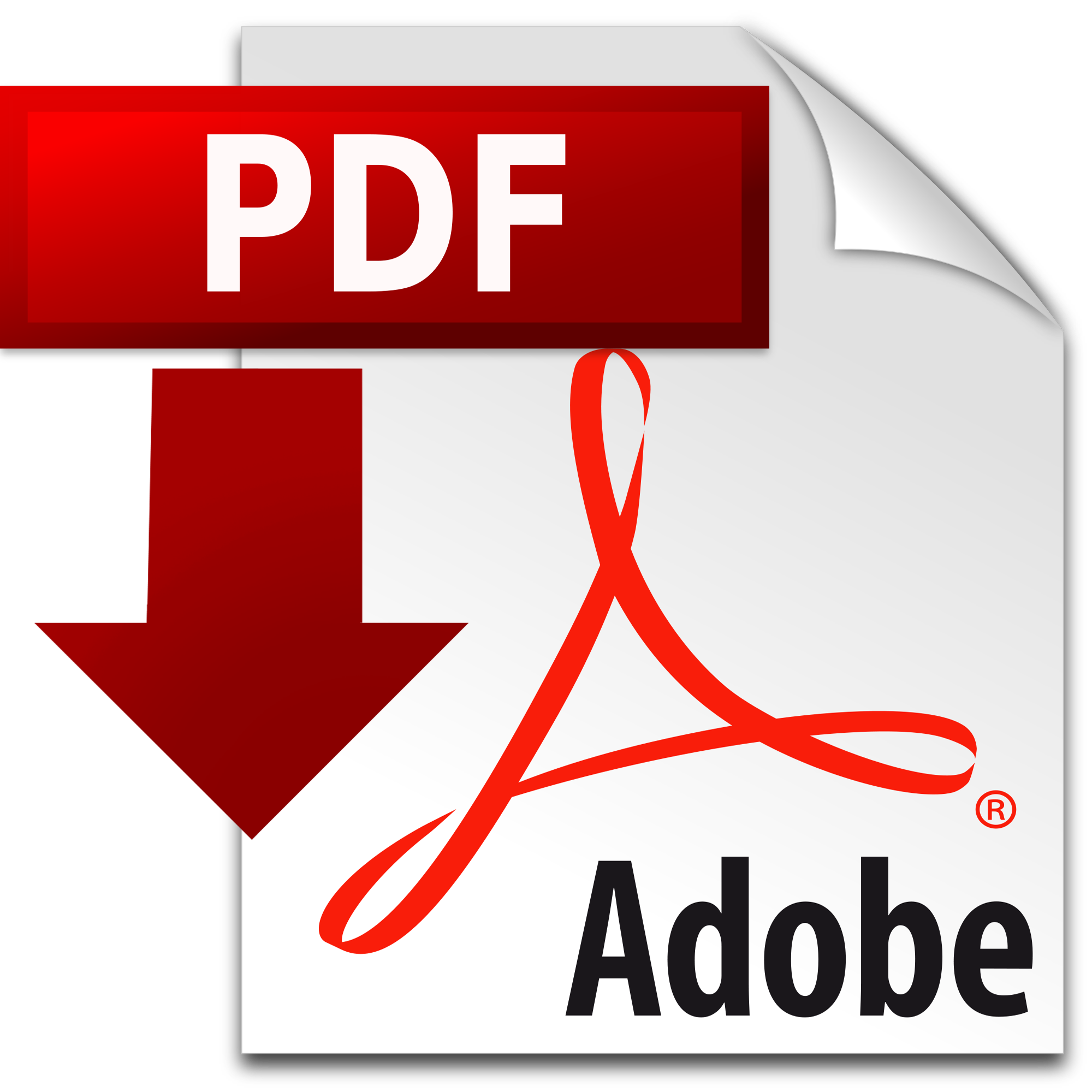
Subsymbolic Computation and the Chinese Room (1991)
In this paper I analyze the distinction between symbolic and subsymbolic
computation, and use this to shed some light on Searle’s “Chinese
Room” argument and the associated argument that “syntax is not
sufficient for semantics”. I argue that subsymbolic models may be
less vulnerable to this argument. I no longer think this paper is very
good, but perhaps the analysis of symbolic vs. subsymbolic computation
is worthwhile. It appeared in The Symbolic and Connectionist Paradigms:
Closing the Gap, edited by John Dinsmore, published by Lawrence Erlbaum
in 1991. [pdf] [philpapers]
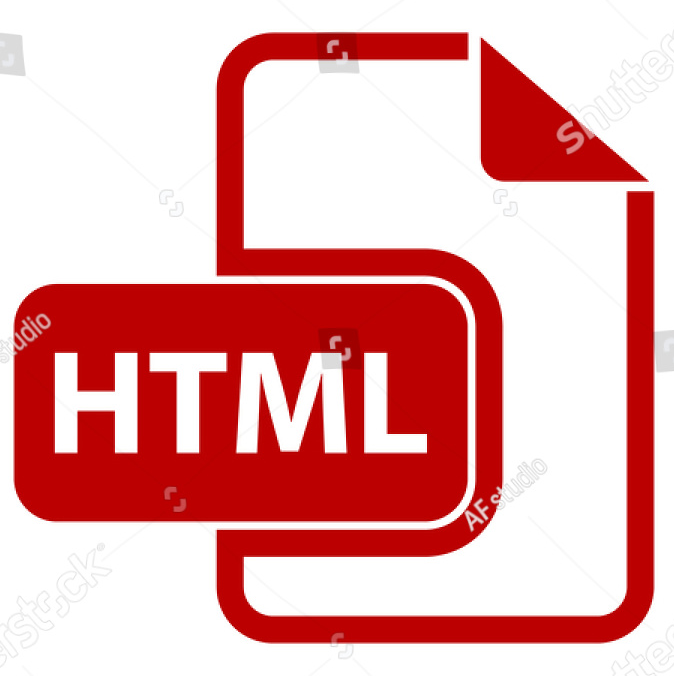
Why Fodor and Pylyshyn Were Wrong: The Simplest Refutation
Proceedings of the 12th Annual Conference of the Cognitive
Science Society, 1990. Hillsdale, NJ: Lawrence Erlbaum. An earlier version of Connectionism and Compositionality: Why Fodor and Pylyshyn Were Wrong. The later version is better. [pdf]
Extended Cognition
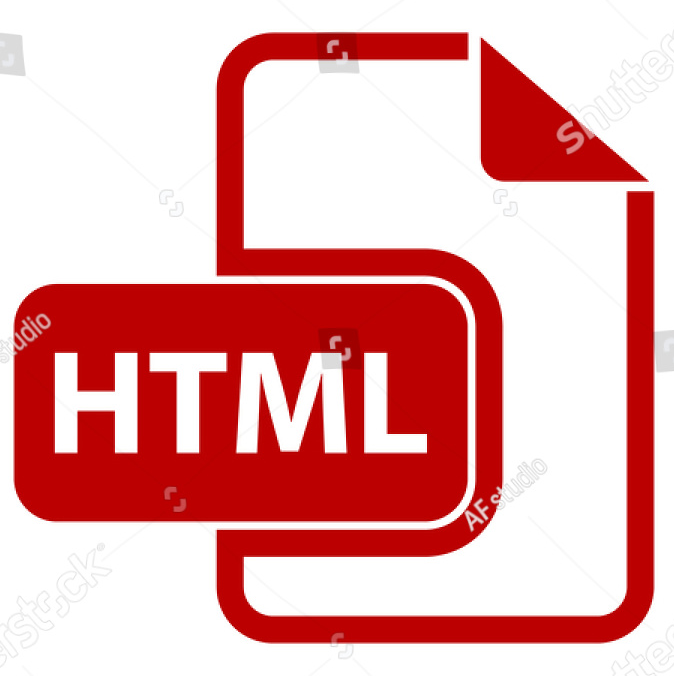
The Extended Mind (1998)
Analysis 58:10-23, 1998. This paper (jointly written with Andy Clark) advocates an “active externalism” where beliefs and other mental states are constituted by objects in the environment coupled to the organism (e.g. someone rearranging Scrabbles tiles on a rack or relying on a notebook as memory, for example). (Reconciling the externalism of this paper with the internalism of “The Components of Content” is left as an exercise for the reader.) This was reprinted in the 1998 The Philosophers’ Annual and by now is easily my most-cited paper. I recently found the first two drafts of this paper with handwritten comments all over them by Andy and me. A couple of follow-up articles are below. [html] [philpapers]
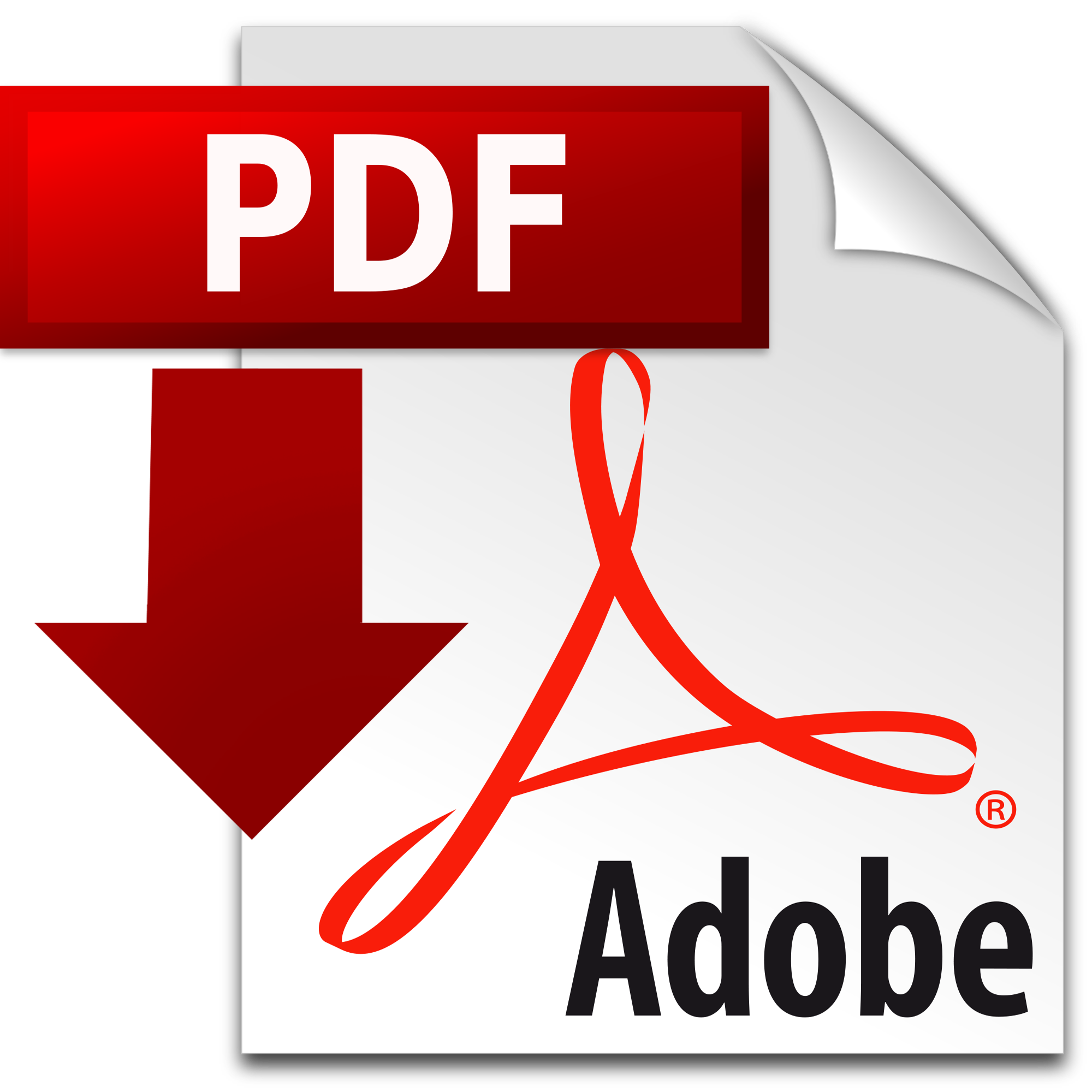
Foreword to Andy Clark's Supersizing the Mind (2008)
A foreword to Andy’s book where I discuss the iPhone as mind-extended and discuss what I think is the strongest objection to the thesis. I go to town on the iPhone idea in this TED talk on the extended mind. [pdf] [philpapers]
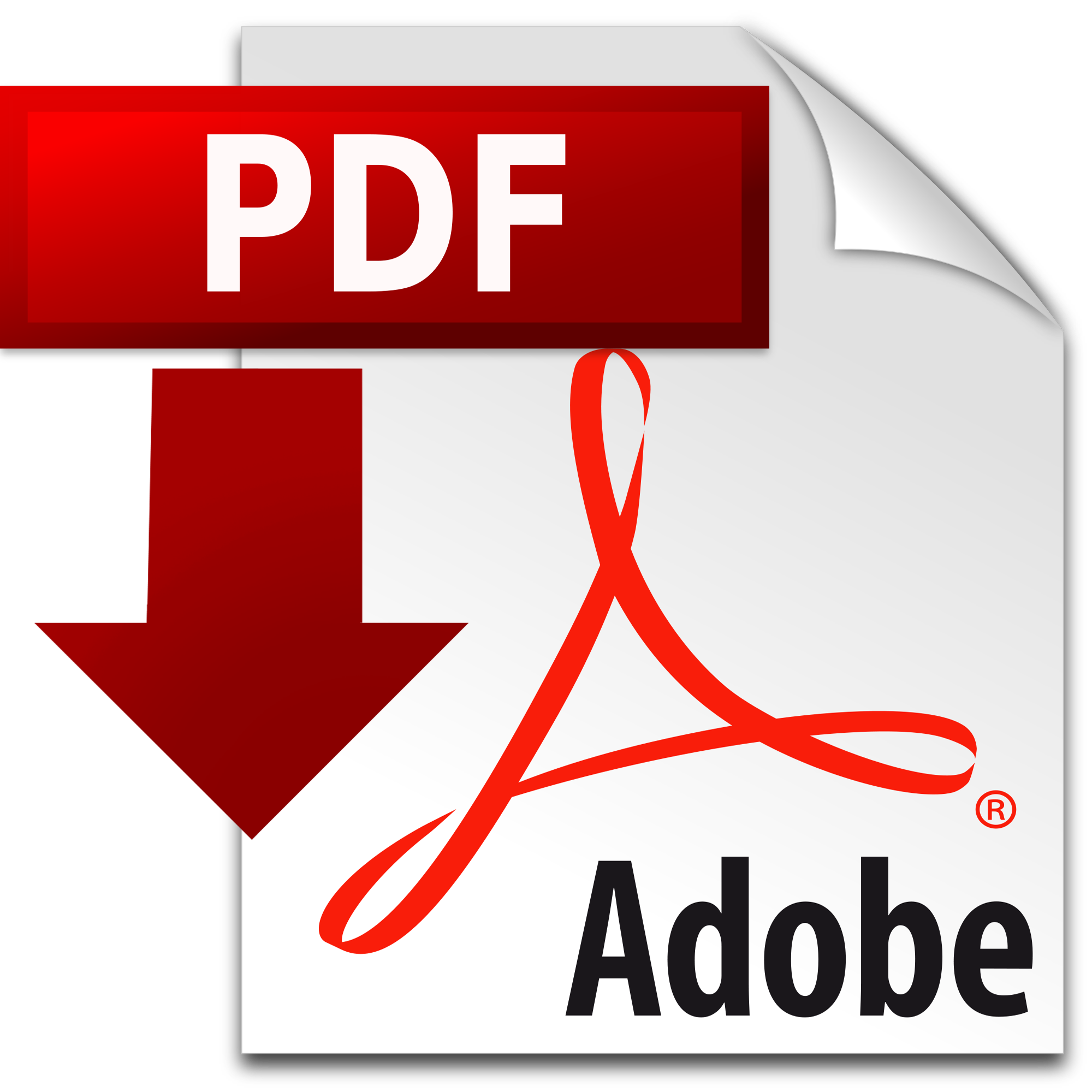
Extended Cognition and Extended Consciousness (2018)
In (M. Colombo, E. Irvine, and M. Stapleton, eds.) Andy Clark and His Critics. Wiley-Blackwell, 2018. A recent piece discussing (i) what is the extended mind thesis, exactly, and (ii) are there cases of extended consciousness, and if not, why not? [pdf] [philpapers]